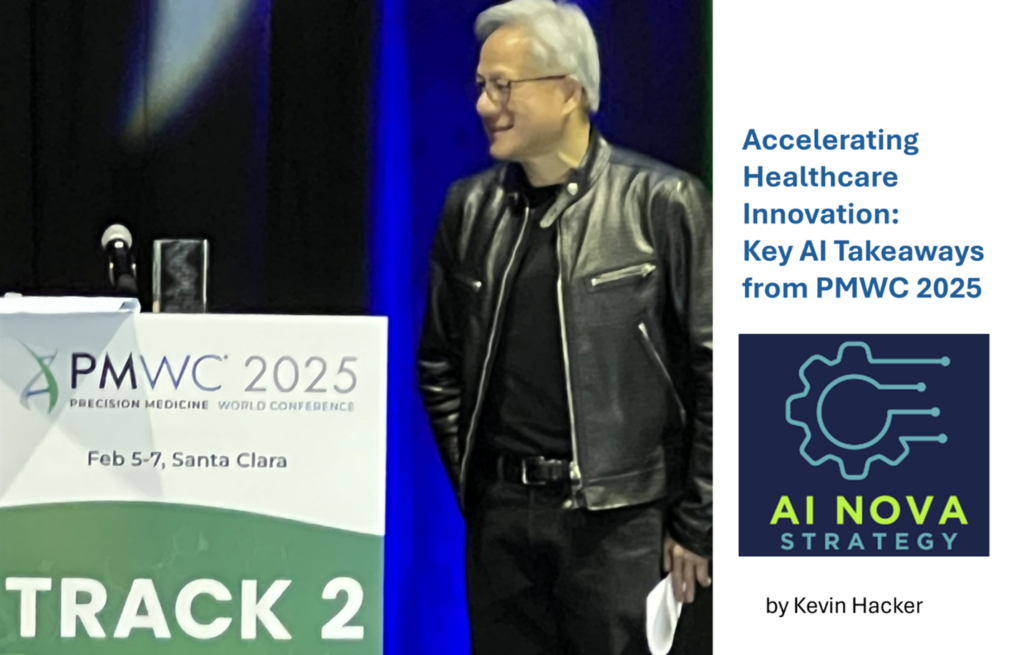
Despite $90 billion being spent annually on cancer treatments, only 10% of drugs are approved for sale by the FDA. The costs of cancer treatments are so high that many patients go bankrupt. We have got to do better. At the conference, many speakers showed how AI tools are improving drug discovery and patient recruitment in clinical trials.
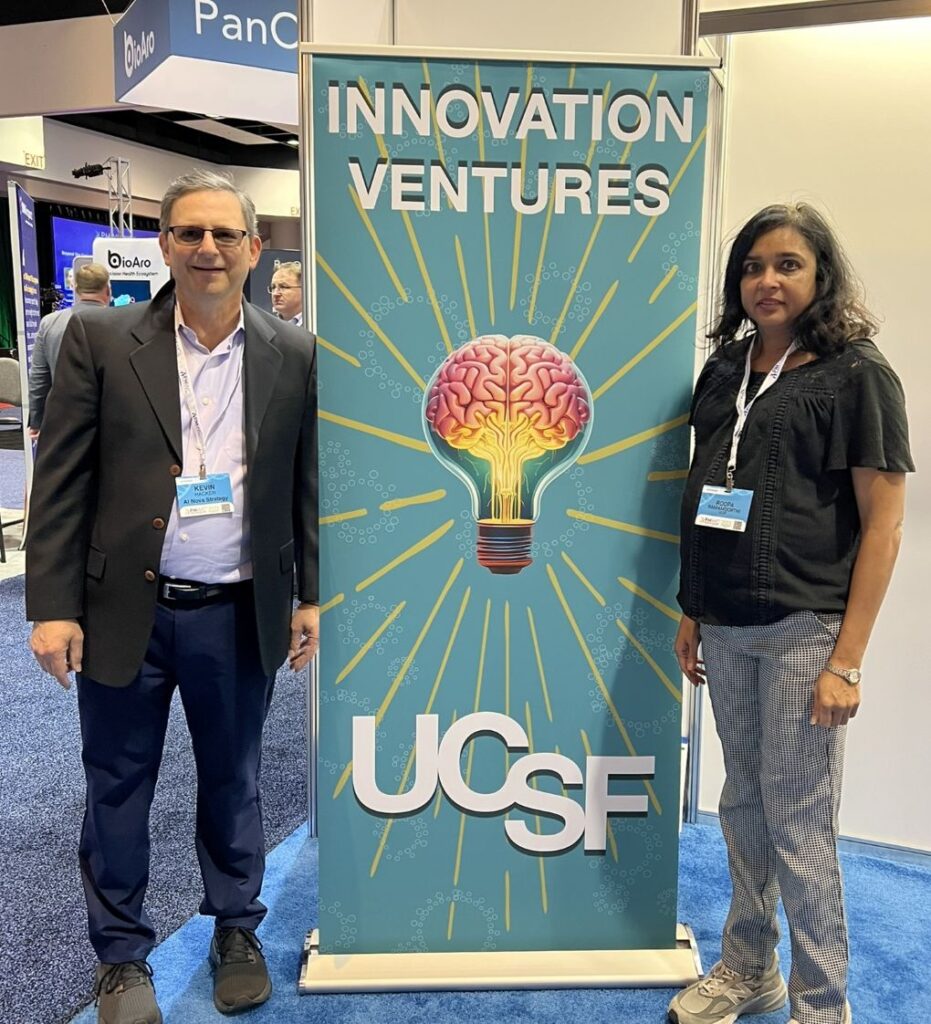
Kevin Hacker, UCSF Catalyst Industry Advisor and Co-founder AI Nova Strategy, and Roopa Ramamoorthi, Director, UCSF Catalyst Program
Shout out to UCSF for co-sponsoring the PMWC.
Key Takeaways
1. Real-world data (RWD) and AI are being integrated into healthcare
AI and RWD, like electronic health records (EHR) and wearables (i.e. iWatches), are being leveraged to improve precision medicine, clinical trials, and patient outcomes. EHR often captures only brief snapshots of patient experiences, leaving significant gaps in understanding the patient journey. Phil Johnson from Evidation Health emphasized the importance of directly engaging patients to collect longitudinal data through wearables, surveys, and molecular data. He demonstrated how its platform uses AI to engage participants, enabling real-time data collection and insights. Johnson also highlighted the importance of ensuring participants’ consent, privacy, and fair compensation for data use, which was a recurring theme, particularly in discussions around wearable devices.
2. AI is being used to predict drug efficacy and identify complex biomarkers that correlate with drug response
AI-driven simulations, such as digital twins, are being used to predict drug efficacy, optimize trial designs, and reduce the time and cost of drug development.
Luca Emili from InSilicoTrials showcased the potential of virtual clinical trials to simulate patient populations and optimize trial parameters, significantly accelerating the drug development process. He highlighted a recent project with Merck where digital twins simulated an entire clinical trial in one day, saving years of research time.
Peter Ellman, CEO of Certis Oncology, discussed using predictive AI to match investigational drugs with specific patient tumor profiles. Certis’ AI platform recently received a U.S. patent, identifies complex gene expression biomarkers, and enables personalized treatment strategies, improving patient outcomes and reducing costs.
3. Advanced AI Models and Knowledge Graphs tools identify disease mechanisms, biomarkers, and drug targets
Knowledge graphs** integrated with large language models (LLMs, such as OpenAI’s GPT) create structured, interpretable insights from diverse datasets.
Janusz Dutkowski from Data4Cure and Paul Rejto from Pfizer demonstrated how these technologies enable researchers to contextualize and compare findings across studies, improving decision-making in drug discovery. Pfizer’s partnership with Data4Cure has resulted in a knowledge graph that integrates omics, clinical, and literature data to provide actionable insights for oncology research. Advances in AI models enable new biology applications, such as protein design, cell state modeling, and multi-omics integration.
Nicolo Fusi from Microsoft Research discussed their work on unified models that combine biological and natural language data, predicting that these models will eventually converge into a single, multi-modal system – a digital representation of humans.
AI is used to power adaptive clinical trials and in silico experiments, dynamically updating trial designs based on real-time data and reducing reliance on traditional static methods.
**Knowledge graphs are structured representations of information that organize data into entities and relationships to capture and model real-world knowledge. They enable machines to understand, reason, and derive insights from interconnected data.
4. Quantum Mechanics-Based AI, multi-omics, and single-cell data are being used in precision medicine
Orly Alter, CSO of Prism AI, highlighted their use of quantum mechanics-based AI to derive interpretable and actionable biomarkers from small, noisy datasets. This approach is being applied to predict patient outcomes and accelerate biomarker discovery.
Integrating multi-omics and single-cell data is critical for understanding disease mechanisms and developing personalized therapies. Yasin Senbaboglu from the Chan Zuckerberg Biohub emphasized using zebrafish and in vitro systems to study dynamic cellular processes. She also highlighted the importance of capturing temporal data (e.g., lineage tracing) to model biological processes better and improve predictive accuracy.
5. AI is revolutionizing medical imaging
AI enables early disease detection, analyzing histopathology data, and integrating imaging with omics data for more comprehensive insights.
Jensen Huang, CEO of NVIDIA, showcased how advancements in GPU-based AI accelerate progress in imaging, genomics, and digital twin applications. NVIDIA’s DGX systems have enabled breakthroughs in AI-powered medical imaging and drug discovery.
The Chan Zuckerberg Biohub demonstrated using self-supervised learning on imaging datasets to uncover new insights into cellular architecture and protein localization. Their work on the OpenCell dataset, which mapped over 1,300 proteins in live cells, highlights how AI can reveal previously unknown subcellular compartmentalization.
6. Industry Collaboration and Data Standardization Are Needed
Collaboration between technology providers, pharmaceutical companies, and regulators is essential for scaling AI applications in healthcare. Examples include partnerships between NVIDIA, Pfizer, and Data4Cure and multi-stakeholder initiatives like the FDA’s Sentinel system.
Efforts to standardize data collection, sharing, and analysis are critical for enabling reproducibility and trust in AI-driven insights. Luca Emili from InSilicoTrials mentioned the FDA’s Good Simulation Practice (GSP) framework, which aims to standardize the use of AI in regulatory submissions.
Summary
The 2025 Precision Medicine World Conference (PMWC) highlighted how AI is transforming cancer treatment and drug development to address the sobering reality that only 10% of drugs reach FDA approval despite $90B annual spending.
The convergence of AI with real-world data creates more efficient pathways for drug discovery and patient care through several breakthrough approaches:
- Digital twins and AI simulations are accelerating drug development while reducing costs.
- Advanced AI models, particularly those combining knowledge graphs with large language models, help decode complex disease mechanisms and identify promising drug targets.
- Quantum mechanics-based AI enables the extraction of actionable insights from small datasets—crucial for rare diseases and personalized medicine.
- Integrating multi-omics data and AI-powered imaging analysis provides unprecedented views into disease progression at the cellular level.
The industry is moving beyond the “more data is better” mindset toward ensuring high-quality data is collected from multi-omics (DNA and RNA sequencing, epigenetics, spatial transcriptomics, and live cell testing). The FDA’s GSP framework and similar guidelines are critical for standardizing AI applications in regulatory submissions.
A key theme throughout the presentations was that AI should augment, rather than replace, human expertise with tools designed to help researchers and clinicians make better-informed decisions.
#AIinHealthcare #PrecisionMedicine #PMWC2025 #DrugDiscovery #AIconsultingfirm