AI NOVA STRATEGY
Our AI implementation roadmap:
- Every engagement begins with our proprietary 50-question survey, precision-tailored for each client. This ensures we provide the best match to system performance and avoid over-investment in AI technology.
- Both bottom-to-top and top-to-bottom interviews provide deep insights into the client’s system challenges, helping us craft a strategic advantage with system thinking and artificial intelligence for businesses of all sizes, from small business AI consulting to enterprise-level firms.
- Collaborative analysis transforms what we learned into a visionary system and AI strategy roadmap, revolutionizing business with AI and paving the way for system and AI-driven business transformation.
- The roadmap presentation delivers game-changing strategies with clear, actionable implementation guidance, offering a system and AI road map for businesses that’s tailored to their unique needs.
- The execution phase is managed with relentless attention to detail, ensuring flawless AI integration across various sectors, including AI biotech consultancy, AI biopharma, and AI fintech business consulting.
- State-of-the-art training programs create AI champions within client organizations, empowering them to leverage artificial intelligence consulting firm expertise for continued growth.
- Our continuous monitoring of AI adoption ensures ongoing optimization and industry-leading results, reassuring businesses that their system and AI deployment will be effective and maintain a competitive edge in the rapidly evolving landscape of AI technology.
*a recent study from the International Data Corporation
(IDC) “The Business Opportunity of AI”
We can help with AI Implementation in your Business Strategy
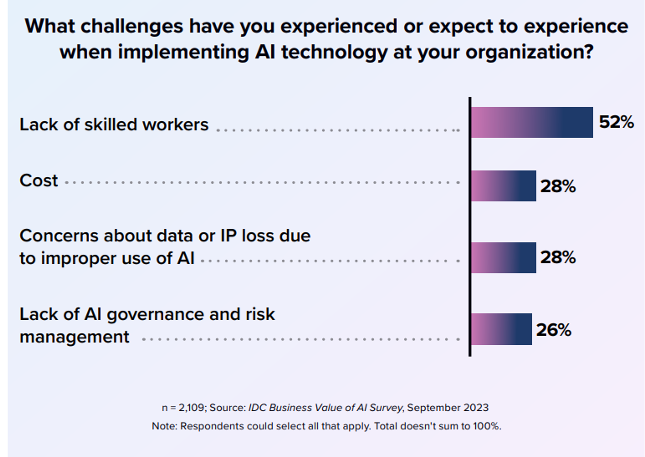
FAQ
What does the partnership process look like?
Our Strategic Partnership Journey:
- Discovery Phase (Week 1)
- Complimentary 30-minute initial consultation
- Custom questionnaire delivery and review
- 15-30 minute follow-up analysis call
- Assessment & Planning (Week 2)
- Initial roadmap development
- 30-minute strategic presentation
- Alignment and feedback session
- Strategic Roadmap Development (Weeks 3-5)
- Detailed, actionable, and scalable strategy development
- Implementation timeline creation
- ROI projections and success metrics
- Implementation Launch (Week 6)
- Comprehensive roadmap presentation
- Written documentation delivery
- Implementation guidance and support plan
- Quarterly review schedule establishment
Investment and specific deliverables will be outlined in our proposal following the initial consultation.
How can AI implementation improve biotech and biopharma research and development processes?
- Drug Discovery and Development
- Cost reduction in R&D processes by up to 25% through AI implementation [1]
- Data Analysis and Insights
- Enhanced processing of complex biological datasets
- Improved pattern recognition in genomic data
- Real-time analysis of clinical trial data
- Better integration of multi-omics data for comprehensive insights [2]
- Process Optimization
- Automated lab processes and workflow optimization
- Quality control and batch consistency
- Supply chain optimization
- Regulatory compliance monitoring [3]
References:
[1] McKinsey (Jan 2024) – “Generative AI in the pharmaceutical industry: Moving from hype to reality”
URL: https://www.mckinsey.com/industries/life-sciences/our-insights/generative-ai-in-the-pharmaceutical-industry-moving-from-hype-to-reality
[2] PMC/NIH Research Article – “Artificial Intelligence in Pharmaceutical Technology and Drug Development”
URL: https://pmc.ncbi.nlm.nih.gov/articles/PMC10385763/
[3] PwC – “Next in pharma 2024: Reinventing for returns”
URL: https://www.pwc.com/us/en/industries/pharma-life-sciences/pharmaceutical-industry-trends.html
What are the emerging trends in AI that will impact the biotech industry in the next 5-10 years?
Here are some futuristic projections:
Advanced Drug Discovery and Development
- AI models will accurately predict drug–protein interactions
- Multi-parameter optimization of drug candidates
- Reduced time-to-market through accelerated discovery processes
- Integration with quantum computing for complex molecular modeling [1]
Personalized Medicine Revolution
- AI-driven patient stratification
- Real-time treatment optimization
- Predictive analytics for patient outcomes
- Integration of genomic data with clinical outcomes [2]
Manufacturing and Process Optimization
- Smart manufacturing facilities
- Real-time quality control and monitoring
- Predictive maintenance
- Supply chain optimization through AI
- Reduced production costs and improved efficiency [3]
Clinical Trial Transformation
- AI-powered patient recruitment and matching
- Real-time trial monitoring and adaptation
- Reduced trial costs and duration
- Enhanced patient engagement and retention
- Better prediction of trial outcomes [4]
Data Integration and Analytics
- Advanced multi-omics data integration
- Real-time analysis of clinical data
- Enhanced disease modeling
- Improved biomarker discovery
- Better understanding of disease mechanisms [2]
Regulatory Compliance and Safety
- Automated safety monitoring
- Enhanced pharmacovigilance
- Streamlined regulatory submissions
- Real-time compliance monitoring
- Improved risk assessment [1]
Emerging Technology Integration
- Combination of AI with:
- Digital twins for process optimization
- Internet of Things (IoT) for real-time monitoring
- Blockchain for data security and traceability
- Advanced robotics for automated labs [4]
Market Impact and Business Models
- Shift towards data-driven decision making
- New partnership models between tech and biotech companies
- Enhanced ROI in R&D processes
- New revenue streams from AI-enabled services [3]
References:
[1] ZS Insights (Feb 2024) – “Pharma trends 2024: Shaping the future landscape”
[2] PwC (2024) – “Next in pharma 2024: Reinventing for returns”
[3] Avenga (Jul 2024) – “Biopharma Trends 2024: AI, Personalized Medicine, And More”
[4] Deloitte Global (May 2024) – “2024 Global Life Sciences Sector Outlook”
How can AI cut healthcare costs and improve patient care?
AI can significantly reduce healthcare costs and improve patient care in several key ways:
Cost Reduction
- Treatment costs can be reduced by up to 50% through AI-powered diagnostics
- Health outcomes can be improved by up to 40% through early detection and intervention
- Streamlined administrative processes reduce operational costs [1]
Clinical Decision Support
- More accurate and faster diagnoses
- Reduced medical errors
- Better treatment planning
- Early detection of health issues
- Personalized treatment recommendations [2]
Operational Efficiency
- Automated administrative tasks
- Optimized resource allocation
- Streamlined workflow processes
- Reduced paperwork
- Better inventory management
- Improved scheduling and patient flow [3]
Healthcare Provider Benefits
- Reduced physician burnout
- More time for direct patient care
- Better work-life balance for healthcare workers
- Enhanced clinical documentation
- Improved communication between care teams [4]
Patient Care Improvements
- More personalized treatment plans
- Better preventive care
- Faster access to medical attention
- Improved patient monitoring
- Better treatment adherence
- Enhanced patient engagement [2]
Healthcare Delivery Optimization
- Remote patient monitoring
- Telemedicine enhancement
- Predictive analytics for patient outcomes
- Better resource allocation
- Reduced hospital readmissions [3]
Data Management and Analysis
- Better use of electronic health records
- Improved population health management
- Enhanced clinical research
- Better disease surveillance
- More effective public health interventions [4]
References:
[1] IBM Think Insights (Jul 2023) – “AI healthcare benefits”
[2] BMC Medical Education (Sep 2023) – “Revolutionizing healthcare: the role of artificial intelligence in clinical practice”
[3] ForeseeMed – “Artificial Intelligence (AI) in Healthcare & Medical Field”
[4] McKinsey & Company (Mar 2020) – “Transforming healthcare with AI: The impact on the workforce and organizations”
What are the key considerations when developing an AI strategy for a biotech company?
Here are the key considerations when developing an AI strategy for a biotech company:
Strategic Alignment and Planning
- Clear definition of business objectives
- Integration with existing R&D processes
- Identification of priority areas for AI implementation
- Development of realistic timelines and milestones
- Resource allocation planning
Safety and Security Considerations
- Development of robust safety protocols
- Implementation of security standards
- Risk assessment and management
- Compliance with regulatory requirements
- Data protection measures
Technical Infrastructure
- Assessment of current IT capabilities
- Cloud computing requirements
- Data storage and processing capacity
- Integration with existing systems
- Scalability considerations
Data Strategy
- Data quality and availability
- Data governance frameworks
- Data standardization
- Privacy compliance
- Data sharing protocols
Talent and Expertise
- Required skill set identification
- Recruitment strategy
- Training programs
- Partnership opportunities
- Knowledge transfer mechanisms
Implementation Approach
- Phased rollout plan
- Pilot program design
- Success metrics definition
- Feedback mechanisms
- Continuous improvement processes
Regulatory Compliance
- Understanding of the regulatory landscape
- Documentation requirements
- Validation procedures
- Audit trails
- Quality control measures
Change Management
- Stakeholder communication
- Training programs
- Cultural transformation
- Process adaptation
- Employee engagement
Partnership and Collaboration
- Identification of potential partners
- Due diligence processes
- IP protection strategies
- Collaboration frameworks
- Resource-sharing agreements
Cost and ROI Considerations
- Budget allocation
- Expected returns
- Investment priorities
- Cost control measures
- Performance metrics
How do you integrate AI solutions with our IT infrastructure and systems?
- Involve all stakeholders early in the process
- Do a data architecture review
- Define KPIs
- Start with pilot projects
What are the potential risks and challenges of integrating AI into our business model?
Technical Risks
a) Data Quality and Management:
- Inconsistent or biased training data
- Data silos and integration issues
- Storage and processing limitations
- Data governance challenges
b) System Integration:
- Legacy system compatibility issues
- API integration complexities
- Performance bottlenecks
- System downtime risks
Operational Risks
a) Implementation Challenges:
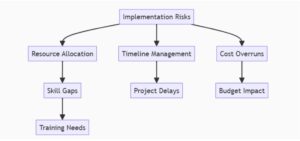
Screenshot
Process Disruption:
- Workflow interruptions
- Employee resistance
- Productivity dips during transition
- Learning curve challenges
Strategic Risks
a) Market Position:
- Competitive disadvantage if implementation fails
- Over-reliance on AI solutions
- Market timing issues
- ROI uncertainty
b) Business Model Alignment:
- Misalignment with core business objectives
- Scalability concerns
- Integration with existing value propositions
- Customer acceptance uncertainty
Regulatory and Compliance Risks
Financial Risks
a) Cost Structure:
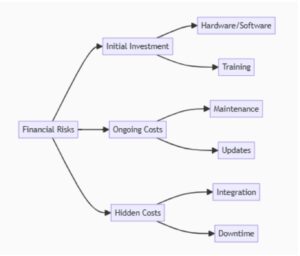
Screenshot
b) ROI Uncertainty:
- Long payback periods
- Unclear cost-benefit ratio
- Unexpected maintenance costs
- Resource allocation challenges
Security Risks
a) Cybersecurity Threats:
- Data breaches
- Model manipulation
- System vulnerabilities
- Intellectual property theft
b) Privacy Concerns:
- Patient data protection
- Proprietary information security
- Cross-border data transfer issues
- Consent management
Human Resource Challenges
a) Skill Gap:
- Limited AI expertise
- Resistance to change
- Cultural adaptation
b) Organizational Impact:
- Role redefinition
- Job displacement concerns
- Team restructuring needs
- Change management challenges
Ethical Considerations
a) Decision-Making:
- Algorithmic bias
- Transparency issues
- Accountability concerns
- Fair use considerations
b) Social Impact:
- Job displacement
- Privacy concerns
- Equal access issues
- Environmental impacts
How scalable are your AI solutions as our company grows?
-
Very.
We help you:
- Build with growth in mind
- Maintain flexibility for new technologies
- Ensure regulatory compliance scalability
- Plan for increased data volumes
How do you ensure your AI strategies remain relevant in the fast-evolving tech landscape?
- Maintain strategic partnerships
- Networking
- Invest in continuous learning
- Have a continuous evolution strategy:
Screenshot
How do you ensure our AI-powered solutions meet regulatory requirements?
We work with you on putting together an AI regulatory requirements plan.
- Regulatory framework integration
- Data management
- Documentation requirements
- Quality assurance
- Regulatory requirement analysis
How can we ensure the security and privacy of our data when implementing AI solutions?
We will:
- For Questionnaire:
– Use Microsoft Forms for the questionnaire
– Include NDA or confidentiality agreement
– Collect only necessary information
– Have straightforward data usage and privacy statement
- For Roadmap:
– Save as password-protected PDFs
– Use secure cloud storage
– Implement version control
– Track who has access
What is the typical cost range for implementing an AI strategy in a biotech company?
Key Cost Factors:
- Company Size
- Data Volume
- Regulatory Requirements
- Integration Complexity
- Existing Infrastructure
[Definitions of small, medium, and enterprise-scale:
Small Scale
– Employee size: 10-50 employees
– Annual revenue: $1M – $10M
– Data volume: <1TB
– Sample processing: <1,000 samples/month
Examples:
– Early-stage biotech startups
– Research-focused small companies
– Specialized diagnostic labs
Medium Scale
– Employee size: 51-250 employees
– Annual revenue: $10M – $100M
– Data volume: 1-50TB
– Sample processing: 1,000-10,000 samples/month
Examples:
– Growing biotech companies
– Mid-sized diagnostic companies
– Contract Research Organizations (CROs)
Enterprise Scale
– Employee size: 250+ employees
– Annual revenue: $100M+
– Data volume: 50TB+
– Sample processing: >10,000 samples/month
Examples:
– Large pharmaceutical companies
– Major diagnostic providers
– Multi-national biotech corporations]
1.Infrastructure Setup
Basic Setup: $50,000 – $100,000
– Cloud infrastructure
– Basic security measures
– Essential data storage
– Basic computing resources
Advanced Setup: $100,000 – $500,000
– High-performance computing
– Advanced security
– Scalable architecture
– Redundancy systems
Enterprise Setup: $500,000 – $2M+
– Custom infrastructure
– Full compliance systems
– Global scalability
– Advanced disaster recovery
2.Software & Licensing (Annual)
Cloud Services (AWS/Azure/GCP): $5,000 – $50,000+/month
ML Platforms: $10,000 – $100,000/year
Specialized Biotech Software: $25,000 – $250,000/year
Security Solutions: $10,000 – $100,000/year
3.Personnel/Training
Data Scientists: $120,000 – $200,000/year per person
ML Engineers: $130,000 – $180,000/year per person
Biotech AI Specialists: $150,000 – $250,000/year per person
Training Programs: $5,000 – $50,000/year
4.Implementation Phases
Phase 1: Pilot Project (3-6 months)
Small Scale: $100,000 – $250,000
Medium Scale: $250,000 – $500,000
Large Scale: $500,000 – $1M+
Includes:
– Proof of concept
– Initial implementation
– Basic integration
– Testing & validation
Phase 2: Full Implementation (6-18 months)
Small Scale: $250,000 – $500,000
Medium Scale: $500,000 – $2M
Large Scale: $2M – $5M+
Includes:
– Full system integration
– Scaled deployment
– Complete training
– Optimization
5.Ongoing Costs (Annual)
Maintenance: 15-20% of implementation cost
Updates & Upgrades: 10-15% of implementation cost
Support: $50,000 – $500,000/year
Compliance & Security: $25,000 – $250,000/year
How can we measure the ROI of implementing AI in our operations?
- Process automation savings
- Research efficiency improvement
- Time-to-market reduction
- Error reduction
What is the estimated ROI on AI implementation?
- Process automation savings: 20-40%
- Research efficiency improvement: 30-50%
- Time-to-market reduction: 15-30%
- Error reduction: 25-45%
Will you help find specialized AI talent or reskill our existing team?
Yes. We have a network of recruiters and vendors and conduct reskilling presentations and workshops.
Why CHOOSE AI NOVA STRATEGY?
We listen to your insights and perspectives.
We draw from extensive life sciences and digital healthcare/fintech to bridge the gap between innovation in these cutting-edge fields to AI implementation.
We propose a detailed and actionable roadmap for AI implementation and help you execute it.
We are centered in Silicon Valley and keep you in the AI technology advancement loop.
We carefully evaluate AI technology and keep you from engaging in the fear of missing out.
We take the security of your information very seriously. Strong ethical considerations are a part of our AI implementation plan.
Elevate Your AI With AI NOVA STRATEGY
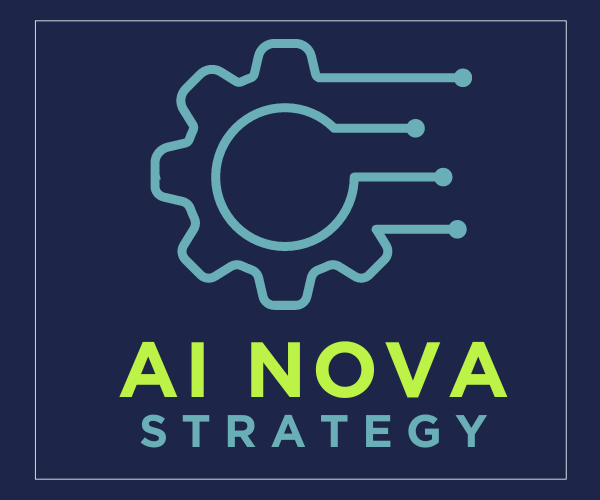
AI NOVA STRATEGY
21701 Stevens Creek Blvd, #744
Cupertino, CA 95014-9998
Ph. 650-400-8761